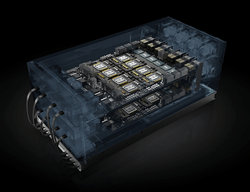
May 30, 2018
By: Michael Feldman
At Taiwan’s GPU Technology Conference this week, NVIDIA founder and CEO Jensen Huang announced the HGX-2, a 16-GPU reference design aimed at some of the most computationally demanding HPC and AI workloads. As a reflection of its tightly integrated design, Jensen characterized the platform as the “the world’s largest GPU.”
HGX-2. Source NVIDIA
According to him, such an architecture can deliver significant price-performance advantages for both high performance computing and machine learning environments compared to conventional CPU-based servers. The V100 processor, upon which the HGX-2 is based, offers both specialized Tensor Cores for deep learning acceleration, as well as more conventional IEEE floating point hardware to speed more traditional high performance computing applications like physics simulations and weather modeling. “NVIDIA’s HGX-2 with Tensor Core GPUs gives the industry a powerful, versatile computing platform that fuses HPC and AI to solve the world’s grand challenges,” Jensen declared.The HGX-2 is actually the design behind NVIDIA’s own DGX-2 server product, which the company launched in March at GTC in California. That product was aimed primarily at machine learning users who wanted to be first in line to use the latest 32GB V100 GPUs in a tightly connected 16-GPU configuration.
Connectivity is the key word here. The DGX-2, and now the HGX-2 upon which it is based, is comprised of 12 NVLink switches (NVSwitches), which are used to fully connect the 16 GPUs. Although that sounds simple enough, the design is a bit more involved. Briefly, the platform is broken up into two eight-GPU baseboards, each outfitted with six 18-port NVSwitches. Communication between the baseboards is enabled by a 48-NVLink interface. The switch-centric design enables all the GPUs to converse with one another at a speed of 300 GB/second -- about 10 times faster than what would be possible with PCI-Express. And fast enough to make the distributed memory act as a coherent resource, given the appropriate system software.
HGX-2 architecture. Source: NVIDIA
Not only does the high-speed communication makes it possible for the 16 GPUs to treat each other’s memory as its own, it also enables them to behave as one large aggregated GPU resource. Since each of the individual GPUs has 32 GB of local high bandwidth memory, that means an application can access 512 GB at a time. And because these are V100 devices, that same application can tap into two petaflops of deep learning (tensor) performance or, for HPC, 125 teraflops of double precision or 250 teraflops of single precision. A handful of extra teraflops are also available from the PCIe-linked CPUs, in either a single-node or dual-node configuration (two or four CPUs, respectively).
That level of performance and memory capacity is enough to run some of the largest deep learning models and GPU-accelerated HPC simulations, without the need for a multi-server set-up. The HGX-2 can also be clustered into larger systems via 100Gbps network inferface cards, but at the expense of being force into a distributed computing model.
Of course, NVIDIA could just have just sold its own DGX-2 to serve these HPC and AI customers. But the company is not really in the system business and is not set up to build and deliver these machines in the kind of quantity it envisions. Making the HGX-2 design available to OEMs and ODM means it can reach a much larger audience, and theoretically sell a lot more V100 GPUs, not to mention NVSwitches.
In particular, making the platform available as a reference design will enable the HGX-2 to be deployed in cloud and other large-scale datacenter environments at volumes and price points that would have been impossible with the $399,000 DGX-2. Lenovo, QCT, Supermicro and Wiwynn have announced plans deliver HGX-2-based servers later this year. In addition, ODMs Foxconn, Inventec, Quanta and Wistron revealed they are designing HGX-2 systems for “some of the world’s largest cloud datacenters.” Those systems are also expected to be available later in 2018.
Although NVIDIA is positioning the HGX-2 as a dual-use platform for HPC and AI, it’s likely that most of the deployments, especially the ones that end up in cloud datacenters, will be primarily running deep learning workloads. That was certainly the case for HGX-1-based installations at Amazon, Facebook and Microsoft. At this point, only a small percentage of HPC work is currently being performed in the cloud and only a fraction of those applications are GPU-enabled.
On the other hand, if Lenovo, Supermicro or one or more of the other system providers manage to sell an HGX-2-based machine to an HPC customer, there is certainly the possibility that it will be used for both traditional simulation work and machine learning. As we’ve reported before, the use of such mixed workflows appears to be on the rise at nearly all large HPC installations and that trend is expected to continue. The major limiting factor here is that not all HPC applications are GPU-ready, but the presence of a super-sized virtual GPU in the HGX-2 makes that limitation easier to overcome.
Looking more broadly, HGX-2 appears to be part of a larger strategy at NVIDIA to provide acceleration technology that is flexible enough to serve both HPC and AI. In that sense, the HGX-2 is really just a server-level extension of the V100 itself. While there is something of a gold rush to develop custom-built AI chips and servers, the supercomputing world would seem to be best served by a unified solution.
Intel briefly flirted with such a product last year, with its AI-tweaked Knights Mill Xeon Phi processor, but the chipmaker has since reversed course. For a time, AMD seemed intent on bringing its CPU-GPU accelerated processing unit (APU) to the datacenter with the Opteron X series, but that product line appears to be stalled. The recently unveiled Prodigy processor from startup Tachyum could theoretically give NVIDIA a run for its money, but even if the technology lives up to its claims, bringing that chip to market and building a software ecosystem around it is going to take many years.
For the time being, that leaves the GPU-maker essentially unchallenged in the unified accelerator space. And that means HPC sites looking to support traditional supercomputing, with a little machine learning on the side, will have a pretty clear idea of which chips they’ll be buying. Which is probably just the way NVIDIA likes it.