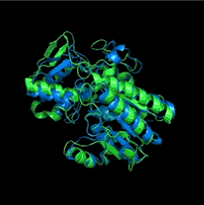
Dec. 3, 2018
By: Michael Feldman
Having moved on from beating up on world champion Go players, DeepMind has developed an artificial intelligence system that just captured top honors in a protein folding prediction competition. Known as AlphaFold, the technology has been two years in the making.
Like its AlphaGo and AlphaGo Zero forerunners, AlphaFold appears to have significantly advanced the state of the art in its chosen field. In this case, the software is aimed at figuring out how proteins are structured based only on the gene sequences that encode them – something that has far-reaching applications in healthcare, agriculture, and environmental protection. According to DeepMind's description of the technology, “[t]he 3D models of proteins that AlphaFold generates are far more accurate than any that have come before—making significant progress on one of the core challenges in biology.”
If you can predict how proteins fold based on their originating gene sequences, you can gain a great deal on insight on how diseases like Alzheimer’s and cystic fibrosis develop, and more to the point, how to prevent them from developing. Such knowledge can also be used to help breed or otherwise genetically modify crops like grains, fruits, and vegetables to increase food production. Likewise, it can be used to engineer microorganisms to safely degrade man-made pollutants like plastic or produce energy biproducts like methane more efficiently.
The challenge of predicting protein structures from genomic data is that DNA only specifies the sequence of the component amino acids. Figuring out how the aggregate structures self-organize into functional 3D structures is incredibly complex. Employing trial and error, it would take longer than the age of the universe to iterate all the possible configurations of a typical protein before reaching the correct structure. To date, most of the progress has relied on laboratory techniques such as cryo-electron microscopy, nuclear magnetic resonance or X-ray crystallography. Such problems are ripe for AI-based solutions.
AlphaFold showcased its protein folding smarts by placing first in the latest Community Wide Experiment on the Critical Assessment of Techniques for Protein Structure Prediction (CASP) rankings, a biannual global competition to gauge protein folding prognostication. And it did so in DeepMind-dominating fashion. According to reporting in The Guardian, AlphaFold predicted the most accurate structure for 25 out of 43 proteins, compared to 3 out of 43 for the number two team.
Source: DeepMind Technologies
In the writeup of the effort, the AlphaFold developers employed a multi-pronged approach using artificial neural networks:
The properties our networks predict are: (a) the distances between pairs of amino acids and (b) the angles between chemical bonds that connect those amino acids. The first development is an advance on commonly used techniques that estimate whether pairs of amino acids are near each other.
We trained a neural network to predict a separate distribution of distances between every pair of residues in a protein. These probabilities were then combined into a score that estimates how accurate a proposed protein structure is. We also trained a separate neural network that uses all distances in aggregate to estimate how close the proposed structure is to the right answer.
Using these scoring functions, we were able to search the protein landscape to find structures that matched our predictions. Our first method built on techniques commonly used in structural biology, and repeatedly replaced pieces of a protein structure with new protein fragments. We trained a generative neural network to invent new fragments, which were used to continually improve the score of the proposed protein structure.
We should expect to see research papers published on the AlphaFold work in the coming months, which presumably will reference the underlying hardware on which the software was run. Given DeepMind’s intimate association with Google, it’s a good bet that the web giant’s custom-built Tensor Processing Units (TPUs) were employed for the research project. In May of this year, Google announced the third-generation TPU, a platform that supplies hundreds of machine learning teraflops per board. Google is saying a multi-rack “pod” of these boards will deliver 100 petaflops.
Although DeepMind developers have been working on AlphaFold for the past two years, the CASP competition is the software’s first public outing. And it’s unlikely to be its last. Mastering protein folding is potentially worth billions of dollars per year to the global pharmaceutical sector, not to mention its auxiliary value to other companies and government agencies that rely on life sciences.
The fact that Alphabet Inc., DeepMind’s corporate master, is looking to reinvent the healthcare business suggests that research projects like AlphaFold will get plenty of backing in the years ahead. In fact, a splinter group known as DeepMind Health, has been established to research and develop AI-based clinical tools for this sector. Combining all that with Google’s commitment to purpose-built AI hardware, the ever-growing base of genomic data, and DeepMind’s relentless refinement of its own software, makes it conceivable that we’ll see commercial applications for this technology is the not-too-distant future.