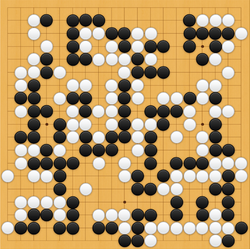
Oct. 23, 2017
By: Michael Feldman
Google’s DeepMind team has reinvented AlphaGo, and this latest iteration was able to resoundingly beat the previous version that defeated Go champ Lee Sedol in 2016, by a score of 100 to 0.
Known as AlphaGo Zero, this new version of the Go-playing AI program uses a form of reinforcement learning to train itself, without any reliance on data from human matches. According to an article posted on the DeepMind site, the program learns the game by repeatedly playing against itself, improving its level of play with each iteration. Here’s the gist of it from the write-up:
The system starts off with a neural network that knows nothing about the game of Go. It then plays games against itself, by combining this neural network with a powerful search algorithm. As it plays, the neural network is tuned and updated to predict moves, as well as the eventual winner of the games. This updated neural network is then recombined with the search algorithm to create a new, stronger version of AlphaGo Zero, and the process begins again.
For each move, the program computes the probability of winning from the choices it has on the board at any given time. As it plays itself through trial and error, this computation becomes more accurate, resulting in increasingly intelligent moves. And it improves itself extremely fast. In just three hours of training, AlphaGo Zero was able to play as well as person who had just learned the game. But after three days of training, the program could play at a super-human level, beating the AlphaGo version that vanquished Lee Sodol last year. And after 40 days of training, AlphaGo Zero was able to vanquish the “Master” version of AlphaGo that defeated world champ Ke Jie earlier this year (although it worth mentioning that the Master program managed to win 11 of those 100 games).
It’s notable that all the previous iterations of AlphaGo were trained using supervised learning based on tens of millions of games played between human beings. The reinforcement learning approach required far fewer games, 4.9 million, to be specific, and needed much less time to train. It’s also noteworthy that the AlphaGo version that defeated Lee Sedol required multiple servers and 48 of Google’s Tensor Processing Units (TPUs), while AlphaGo Zero managed to outsmart its predecessor using a single machine equipped with just 4 TPUs.
The big takeaway here is that this approach, without the benefit of any training data, resulted in a significantly smarter Go player than AI programs that were able to analyze reams of human matches. As the DeepMind article points out, if similar techniques could be used to attack other structured problems, like protein folding or materials design, it could result in breakthroughs across many domains. And removing the impediment of relying on large datasets, means this technology has the potential to be much more broadly applied.