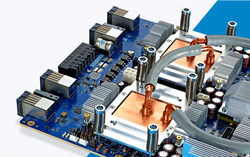
May 11, 2018
By: Michael Feldman
At the Google I/O conference this week, CEO Sundar Pichai announced TPU 3.0, the third iteration of the company’s Tensor Processing Unit, a custom-built processor for machine learning.
Sundar Pichai introducing third-generation Tensor Processing Unit at Google I/O conference.
In truth, Google revealed very little about the new device. Pichai said that a multi-rack TPU 3.0 pod would have more that eight times the performance of a second-generation TPU pod. That’s a little misleading though, since from the images shown at the conference, the TPU 3.0 pod looks to have twice as many racks (8) and twice as many TPU boards per rack (32) as its predecessor. That extra density forced Google to adopt liquid cooling for these new racks.
Taking into account the greater number of TPUs in the new pods, that would mean TPU 3.0 is about twice as powerful as its predecessor, which is nonetheless an impressive performance jump in just a little over a year. That would mean the new TPU can deliver something north of 360 teraflops. Overall, Google is promising more than 100 petaflops of machine learning performance per pod. So training that previously the better part of a day on the older TPUs will be able to execute in less than an hour.
TPU 3.0 Pod.
Again, from the images presented at the conference, the TPU 3.0 board is comprised of four chips, which is the same as the second-generation boards. However, the new boards have twice the capacity of high bandwidth memory (128 GB) as the previous hardware. That will make the hardware more practical for larger models that demand more data. That said, given the concurrent two-fold increase in flops, the bytes to flops ratio will essentially be the same as it was before.
In the machine learning realm, comparing these TPUs to GPUs is difficult. Even ignoring the fact that each TPU board contains four chips, the flops themselves are not equal. The TPU uses something called “brain floating point” numbers, a 16-bit format with a larger exponent and smaller mantissa that a regular IEEE half-precision floating point numbers. By contrast, the Tensor Cores in an NVIDIA Tesla V100 GPU uses more conventional 16-bit and 32-bit floating point values, albeit with specialized multiply-and-accumulate hardware. A V100 can deliver 125 teraflops per GPU using these built-in Tensor Cores.
The real proof is in application performance and Google has done a lot of work in this area on the software side to extract more of the flops and reduce the run-times for training. At the conference, they demonstrated impressive performance using their second-generation TPUs on a variety of applications, including image recognition and object detection, machine translation and language modeling, speech recognition, and image generation.
As we recently reported, Google has added NVIDIA V100 GPUs to its public cloud, but the company seems a lot more interested in developing its TPU cloud offering these days. That TPU service was launched back in February with second-generation hardware, and so far users can only rent a single TPU board at a time. From what was discussed at the conference though, Google will soon make entire TPU pods available, which will mean customers will be able to do heavy-duty training with this cloud service. And at some point in the not-too-distant future, the company will open up the TPU 3.0 devices to the public, enabling machine learning customers to tap into supercomputer-level performance.