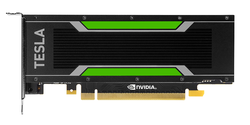
Sept. 12, 2016
By: Michael Feldman
NVIDIA has unveiled the Tesla P4 and P40, two new GPUs aimed at the very latest AI machinery. The processors are based on the company’s Pascal architecture and incorporate new features aimed at deep learning inferencing work in areas like image and speech recognition, language translation, and recommendation engines. The announcement was made at NVIDIA’s GPU Technology Conference taking place in Beijing, China this week.
The new GPUs are the latest entrants in a quickly evolving AI market, where speed and efficiency are paramount. Case in point: when Baidu moved to its second-generation Deep Speech platform for recognizing English and Mandarin, it required 10 times the computational performance of its original technology. Deep Speech 2 supported 12 thousand virtual neurons and 100 million parameters – two and a half times the number of neurons and four times the number of parameters, respectively, as their previous speech recognition platform. For their efforts, Baidu was able to demonstrate an speech recognition error rate of just 2.5 percent, which beats out the 6 percent error rate attained by humans.
According to Marc Hamilton, Vice President Solution Architecture & Engineering at NVIDIA, they realized early on that the deep learning market would need something better than just brute force hardware improvements, a la Moore’s Law, to keep up with customer demands like Baidu. What was required, says Hamilton, was design innovation in the silicon as well as targeted software support.
“We saw this coming many years ago,” he told TOP500 News. “If you compare the products we have announced with the Pascal family – the P100 all the way to the P4 – we’re 40 to 60 times faster than where we were three years ago with the Kepler GPUs.”
That foresight has paid off, with NVIDIA currently in the driver’s seat with the deep learning customer base. Hyperscale companies, like Google, Baidu, Amazon, and Microsoft are rapidly building up their infrastructure for these capabilities, and the vast majority of that hardware uses NVIDIA GPUs, at least for training the neural networks. At the moment these companies appear to have an insatiable appetite for greater performance and capabilities as they build deeper and more complex networks in order to deliver greater accuracy.
Where NVIDIA is not as dominant is the less compute-intensive inferencing part of the work, that is, using the trained networks on a day-to-day basis. Although inferencing must often be accomplished in real-time or at the very least in a highly interactive manner, it requires a trillion times less computation than training a neural network. Thus, even lowly CPUs can be employed for such tasks. GPUs however, can offer many advantages.
That’s where the P4 and P40 come in. Basically, these latest GPUs, are the Pascal versions of the M4 and M40, the Maxwell-based deep learning GPUs released in 2015. But that’s somewhat of an oversimplification. Compared to their Maxwell predecessors, the P4 and P40 are more precisely tailored for deep learning workloads, and specifically for inferencing neural networks. And most of the performance gains attributed to the newer silicon are based on these design changes rather than the architectural upgrade you get with Pascal.
In particular, NVIDIA dropped support for 64-bit double precision floating point (FP64) arithmetic in the P4 and P40 and replaced it with support for 8-bit integer (INT8) arithmetic. Not only does INT8 make a lot more sense for inferencing, but it also uses less memory space and bandwidth than its 64-bit, or even 32-bit counterparts. Essentially, you can execute a lot more instructions with the same number of transistors.
Source: NVIDIA
The P4 GPU module is the smaller of the two, a half-length, half-height card intended for deployment in existing server infrastructure in hyperscale datacenters or elsewhere. The idea here is that if these servers are already doing things like image or speech recognition, even as a part-time gig, they could do much more of it with a P4 at their disposal. At 50 to 75 watts, this GPU can easily slot into an existing web server and turn it into an inferencing speed demon.
The P4 delivers 22 teraops (TOPS) of INT8 and 5.5 single precision teraflops. It comes with 8 GB of GDDR5 memory, which can deliver data to the GPU at up to 193 GB/sec. On paper, it’s about twice as fast as the older M4, not including the additional INT8 capability. It also comes with a video decode engine and two video encode engines, since a good deal of inferencing work is video-based.
The rationale behind the P4 is to offer maximum energy efficiency for these workloads for large-scale deployments. According to NVIDIA, the GPU is 40 times better in delivering images per second per watt than an Intel Xeon CPU. It’s also about four times better than the previous-generation M4 GPU in this area, again, mainly due to the fact that the INT8 support allows for much greater throughput than the M4’s 32-bit floating point (FP32) math.
The P40 is somewhat of a different animal. Although it is also optimized for inferencing, it’s powerful enough to do training as well. Overall it’s more than twice as fast as the P4, delivering 47 INT8 teraops and 12 single precision teraflops. It does that with just 50 percent more cores than the P4, but at a faster processor clock. At 250 watts it’s very much a specialist play, targeted to servers dedicated to deep learning, and customers looking to get the highest throughput possible per box.
A prime candidate for the P40 is Facebook, the first hyperscaler to employ NVIDIA’s M40 GPU. Those were used in Facebook’s “Big Sur” platform, which they open sourced under umbrella of the Open Compute Project. Replacing those M40s with P40s would could significantly speed those workloads. NVIDIA has documented an 8-fold performance increase from the M40 to the P40 on GoogleLeNet, a convolutional neural network (CNN) for vision and pattern recognition, and AlexNet, another CNN used for image recognition.
For dedicated training purposes, the Tesla P100 is still the go-to GPU. Unlike, its P4 and P40 siblings, the P100 comes with NVLink, an extremely fast communication interconnect for multiple GPUs in a server, as well as second-generation high bandwidth memory (HBM2), for speedier data access. Neither of the GPUs announced this week have those features, since for inferencing such features would be overkill. It is worth noting though that the P40 does come with 50 percent more local memory (24 GB) in the form of GDDR5 than the P100 (16 GB), even though it operates at about half the bandwidth of the HBM2. Also, the P40 tops out at 250 watts, which is 50 less than that of the P100.
In any case, this is all good news for companies racing to incorporate deep learning into their products and services. With these more targeted designs, NVIDIA is able to deliver applications speed-ups over and above what could be achieved with Moore’s Law alone. And with Intel looking to take a bite out of NVIDIA’s deep learning revenue stream with the upcoming Knights Mill Xeon Phi, and customized deep learning chips in the offing, NVIDIA needs to find ways to stay ahead of the competition.
Along with with the P4 and P40 unveiling, NVIDIA announced a couple of deep learning software offerings: TensorRT and the DeepStream SDK. TensorRT is a library designed to deliver much faster response times when inferencing neural networks. The DeepStream SDK supports deep learning video analytics, decoding and preprocessing video data to pass on to an inferencing engine. Both are meant to be used in conjunction with the new Pascal GPUs.
The P40 card is scheduled to ship in October, with the P4 following in November. Servers equipped with the new GPUs will initially be available from a handful of OEMs, including Dell, HPE, Lenovo, and Inspur, in addition to ODMs Inventec, Quanta Computer and Wistron.