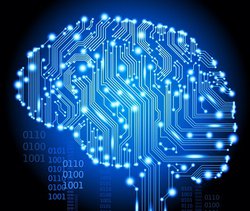
March 31, 2017
By: Michael Feldman
In November 2015 when NVIDIA CEO Jen-Hsun Huang proposed that “machine learning is high performance computing’s first killer app for consumers,” there was only sketchy evidence to back up that claim. Today though, it looks like the NVIDIA chief was just a little ahead of his time.
He went on to say that “supercomputing technology is in the process of extending well beyond supercomputing itself. These advancements are in the process of revolutionizing consumer applications, cloud services, the auto industry and autonomous machines.”
When Huang spoke those words at the 2015 Supercomputing Conference (SC15) in Austin, Texas, NVIDIA stock was trading at around $30 per share. Currently, it’s selling at over $100 per share, primarily driven by the company’s rapid penetration into the expanding machine learning market. As it stands today, NVIDIA’s latest Tesla P100 GPU is the go-to processor for training neural networks in datacenters large and small, while its Tegra and DRIVE products are racking up wins in AI-based applications in autonomous vehicles.
Of course, the effect of machine learning on the HPC industry goes much deeper than NVIDIA’s fortunes. Just about every HPC vendor has devised some sort of machine learning/AI strategy. To review some of the major players: Intel is moving aggressively to challenge NVIDIA GPUs with its upcoming Xeon Phi and Nervana-based product lines; IBM is continuing to expand the skillset and capability of its Watson AI platform; and Cray is fleshing out a strategy that merges its big data and machine learning ambitions with its supercomputing platforms. Even Mellanox has outlined how its interconnect products are being used to accelerate deep learning applications for some of its biggest customers.
Training neural networks is the most compute-intensive activity in many of these workflows, and is therefore the one most demanding of HPC technologies like high-end GPUs, 3D memory, and high performance interconnects. Training these networks is a major activity for the largest web companies in the world, especially Google, Microsoft, Amazon, Apple, and Baidu. They mostly use it to power consumer-facing services that nearly everyone is familiar with: web searching, image recognition, natural language processing, traffic navigation, personalized product selection, and language translation. Since these companies now depend upon high performance computing for their day-to-day operations, there is a huge potential for HPC vendors to expand their customer base beyond their traditional government and commercial users.
The rosy outlook for consumer-oriented AI is borne out by a InsideSales.com study. According to survey data they collected, 54 percent of US consumers already use AI in their daily lives, most commonly for travel, entertainment, navigation, and more recently AI-enhanced personal assistants. A much smaller percentage, 46 percent, have used AI at work. While that covers a large swathe of people, the annual revenue for this space is still relatively small, amounting to just $644 million in 2016. According to AI analyst firm Tractica though, the market is expected to double this year and then grow to $36.8 billion by 2025.
That would suggest that AI will extend well beyond its consumer base and the web giants who serve them. In particular, this technology is poised to penetrate the enterprise. A recent report in Forbes cited a study by Tata Consultancy Services (TCS), which revealed that 84 percent of the company executives they survey see AI as “essential” to competitiveness, and half see the technology as “transformative." Further, about one third of these executives think that their sales, marketing, and customer service activities will use AI, while about one fifth believe the biggest impact of AI will be in the areas of finance, strategic planning, corporate development and human resource functions. These numbers far exceed those for traditional HPC when applied to the broader commercial landscape.
It likely that AI and machine learning will soon be integrated into all data analytics/big data applications as we know them today. At the same time, more advanced AI will create completely new applications, such as autonomous vehicles and ubiquitous digital assistants. While these personal applications may not need HPC technologies per se, there is every reason to believe they will be backed by high performance infrastructure located in a datacenter. For example, self-driving cars are not just standalone robots that replace human drivers. These vehicles will be networked with one other across a server-powered backbone so that they can operate more intelligently and efficiently than would be possible by an isolated machine.
The HPC user community is also trying to figure out how to apply machine learning to its traditional physical model-based domains. For example, Microsoft researchers have looked at using machine learning to provide accurate weather forecasts without the need for any meteorological simulations. IBM, meanwhile, is exploring ways to combine physical and machine learning models to deliver more reliable weather predictions. Other data-rich HPC domains, such as drug discovery, financial analytics, manufacturing, and oil & gas exploration, are also ripe for machine learning technology, either to replace specific applications or to augment them.
Supercomputers are also increasingly being used to run AI workloads not associated with traditional HPC applications. One of the most notable recent examples was the poker-playing AI known as Libratus, which used the Bridges supercomputer at the Pittsburgh Supercomputing Center (PSC) to decisively beat some of the top players in the world. While the demonstration just involved a game, the achievement illustrated that AI could be applied to the kinds of ambiguous problems humans encounter every day, where data is often missing or misleading. That includes applications in business negotiations, intelligence analysis, and medical diagnosis, where people often rely on hunches and intuition to come to a decision.
As a result, machine learning and AI are going to transform nearly every application domain, as well as create entirely new ones. The fact that this software will depend on high performance computing machinery means that the community can hitch its wagon to this technology and, for the first time in its history, ride HPC into the mainstream. As Jen-Hsun Huang put it: “a lot is about to change.”