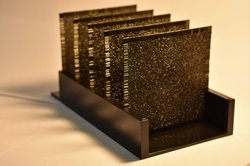
Aug. 7, 2018
By: Michael Feldman
A team of engineers and computer scientists at the University of California, Los Angeles (UCLA) has built a gadget that can detect and classify images at the speed of light.
The device, which the researchers have dubbed a “diffractive deep neural network,” is able to use light bouncing off an object to identify it almost instantaneously. It’s built from a series of polymers wafers with uneven surfaces that is able diffract light in such a way as to enable a trained network to recognize what it’s “seeing.”
Each wafer, which is eight square centimeters, is made up of tens of thousands of artificial neurons in the form of tiny pixels. When a number of wafers are grouped together, they are able to act as an “optical network.” According to the UCLA press release, “[t]he network is able to identify a because the light coming from the object is mostly diffracted toward a single pixel that is assigned to that type of object.” Aydogan Ozcan, the study’s principal investigator, says the device can be thought of as a maze of glass and mirrors in which light entering the maze bounces around and eventually exits at particular locations based on the shape of the object.
Before it can be used, the optical network must be trained beforehand to recognize specific types of objects, in this case, using conventional deep learning techniques. Once that’s done, no additional software is required to identify (inference) the objects. More importantly, no energy is required, since the device only makes use of diffracted light. And here’s the best part: the polymer wafers can be created with a 3D printer from inexpensive materials. The device built by Ozcan’s team could be reproduced for less than $50.
“This work opens up fundamentally new opportunities to use an artificial intelligence-based passive device to instantaneously analyze data, images and classify objects,” said Ozcan. “This optical artificial neural network device is intuitively modeled on how the brain processes information. It could be scaled up to enable new camera designs and unique optical components that work passively in medical technologies, robotics, security or any application where image and video data are essential.”
One obvious application is self-driving automobiles, where the technology could be used to replace the car’s camera and the on-board computer used for the detection of objects – a stop signs, pedestrians, other vehicles, and so on. Not only would this reduce the latency needed for such processing, it would do so without the need for additional energy.
That’s assuming, of course, that the device can match the accuracy of neural networks using more conventional deep learning hardware and software. In the work done so far, the researchers demonstrated the device could accurately identify handwritten numbers, as well as items of clothing.
The research is described in a study published in Science on July 26.
Image: Optical neural network. Source: Ozcan Research Group/UCLA