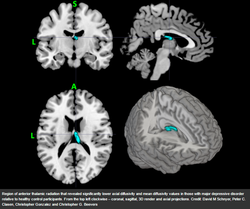
March 31, 2017
By: Michael Feldman
David Schnyer, a cognitive neuroscientist at The University of Texas at Austin has developed machine learning software to diagnose depression based on MRI brain scans. The software was trained on Stampede, a 9.6- petaflop supercomputer housed at the Texas Advanced Computer Center (TACC).
According to an analysis by Schnyer (University of Texas at Austin) and his research cohorts, Christopher Gonzalez (University of California, San Diego), Peter Clasen (University of Washington School of Medicine), and Christopher Beevers (University of Texas at Austin), the software is able to “classify individuals with major depressive disorder with roughly 75 percent accuracy. Depression affects about 6.7 percent of the population in the US.
Source: Texas Advanced Computing Center
In the TACC announcement, the machine learning training setup is described as follows:
“The type of machine learning that Schnyer and his team tested is called Support Vector Machine Learning. The researchers provided a set of training examples, each marked as belonging to either healthy individuals or those who have been diagnosed with depression. Schnyer and his team labelled features in their data that were meaningful, and these examples were used to train the system. A computer then scanned the data, found subtle connections between disparate parts, and built a model that assigns new examples to one category or the other.”
As training data, the researchers used a type of MRI scan known at diffusion tensor imaging (DTI). With these scans, they are able to quantify the integrity of white matter pathways in the cerebral cortex, which exhibit different patterns in healthy and depressed individuals. “Not only are we learning that we can classify depressed versus non-depressed people using DTI data, we are also learning something about how depression is represented within the brain," said Beevers. "Rather than trying to find the area that is disrupted in depression, we are learning that alterations across a number of networks contribute to the classification of depression."
The granularity of the measurements and the size of the problem required a machine learning approach, as well as a powerful HPC system, in this case, Stampede. According to Schnyer, this type of work isn’t possible to do on desktops; it requires the type of supercomputing resources typically found at centers like TACC.
As encouraging as these early results are, Schnyer says the software is not yet ready to be used in a clinical setting. The 75 percent accuracy rate was derived from a relatively small dataset of brain scans of less than 100 individuals (52 participants with depression, 45 without). He thinks they need to draw on a larger population, as well as incorporate other types of information, such as genomics data.
To help realize that goal, the researchers are planning to expand their study to include several hundred individuals that have been diagnosed with depression or a related disorder. They also intend to employ Stampede 2, TACC’s future 18-petaflop supercomputer, which is scheduled to be installed this summer. The researchers are hoping the increased processing power of the new machine will allow them to chew through a lot more data and achieve better accuracy.
"This is the wave of the future," Schnyer said. "We're seeing increasing numbers of articles and presentations at conference on the application of machine learning to solve difficult problems in neuroscience."