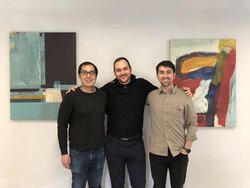
Feb. 7, 2018
By: Michael Feldman
Chip startup Lightmatter has received an infusion of $11 million from investors to help bring the world’s first silicon photonics processor for AI to market. Using technology originally developed at MIT, the company is promising “orders of magnitude performance improvements over what’s feasible using existing technologies.”
Based in Boston, Massachusetts, Lightmatter was founded in September 2017 by Nicholas Harris, Darius Bunandar, and Thomas Graham. Harris and Bunandar are the techies of the trio and occupy the roles of Chief Executive/President and Chief Scientist, respectively, while Graham, the business major, is the company’s Chief Operating Officer. In May of last year, the Lightmatter team won Harvard’s President’s Innovation Challenge and MIT’s $100K Entrepreneurship Competition, which together brought in $175,000 – enough money to jumpstart the company.
Lightmatter executive team. Left to right: Darius Bunandar, Thomas Graham, and Nicholas Harris
The technology was developed over a four-year span at MIT’s Quantum Photonics Laboratory. MIT owns some of the original patents related to the technology, and has licensed them to company to spur further development. While a PhD student there, Harris constructed a prototype at the lab as part of his doctoral thesis.
Like other programmable optical processors, the Lightmatter chip uses light, rather than electrons, as the basis for its processing. Not only does that circumvent the computational speed limit associated with electronic transistors, it does so using just a fraction of the energy. However, the Lightmatter chip is not a general-purpose processor. Instead its silicon photonic circuitry is built to only perform matrix multiplications – the critical computations used by deep learning applications.
A conventional processor is used to host the application, that is, perform the less computationally-demanding part of the application, while offloading the required matrix math to the photonics chip. In that sense, it uses the same host-accelerator paradigm of CPU-GPU platforms. That simplifies the Lightmatter hardware significantly, allowing the design to rely on relatively simple nanophotonic circuits. Given the current immaturity of building nanophotonic structures with CMOS technology, that’s a huge advantage.
“For decades, electronic computers have been at the foundation of the computational progress that has ultimately enabled the AI revolution, but AI algorithms have a voracious appetite for computational power,” said Harris in the company’s first press release. “AI is really in its infancy, and to move forward, new enabling technologies are required. At Lightmatter, we are augmenting electronic computers with photonics to power a fundamentally new kind of computer that is efficient enough to propel the next generation of AI.”
The trickiest part is converting the electron signals to optical signals when doing the electronics-photonics handoff, which requires that binary values be converted into photons. Larger values translate into more photons, that is, brighter light. Once the conversion is complete, the computation can be parallelized by splitting the light into different channels and recombining them, according to the desired computation. Thanks to the use of photons as the computing medium, these transformations can happen at light speed.
The design was tested last summer using a prototype built with an array of 56 programmable Mach–Zehnder interferometers implemented as a silicon photonic integrated circuit. In the demonstration, recorded vocal sounds were used to train a neural network for vowel recognition. The photonic chip was used to decipher vowels based on the trained network. To perform this recognition, the Lightmatter prototype was only moderately accurate (about 75 percent) compared to results on conventional hardware (about 90 percent), but for a first try, it was suitably impressive.
To build a more powerful processor and do more extensive testing will require additional effort, which is why the Series A funding announced this week was good news. That $11 million investment round, which was led by Matrix Partners and Spark Capital, is pretty much on par for early stage AI startups nowadays, but only a fraction of what will be needed to get a commercial product out the door.
Two older AI chip startups – Graphcore and Wave Computing – have already pocketed more than $100 million apiece from investors. Graphcore has received a total of $110 million after three funding rounds, while Wave Computing has collected 117.3 million after four rounds. Cerebras Systems, another such startup, has taken in $52 million after two rounds. All three use conventional electronics for their chips.
Which is to say Lightmatter has a long way to go before it can take on its competition, much less AI behemoth NVIDIA. Stan Reiss, general partner at Matrix Partners, admits there is “still a tone of technical risk,” but he believes the advantage of implementing AI algorithms with silicon photonics represents an enormous opportunity.
“They will need to scale up their prototype, build out the rest of the system, write the software, build the tools,” writes Reiss. “They may be delayed, and there is of course a chance of failure. But if they succeed, not only will they build a disruptive AI processor, they will have started the optical computing revolution.”