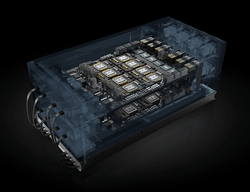
Nov. 23, 2018
By: Michael Feldman
NVIDIA continues to rack up wins with HGX-2, the company’s 16-GPU server platform for accelerating artificial intelligence, analytics, and HPC.
HGX-2 is the NVIDIA’s latest GPU-dense server platform, providing 16 V100 Tesla GPUs glued together with NVSwitch, a high-performance fabric that enables multiple GPUs to operate as a unified resource.
At the GPU Technology Conference (GTC) in China earlier this week, it was announced that internet giants Baidu and Tencent are deploying HGX-2 systems as the basis for new machine learning/deep learning capabilities. The servers will be used both internally to expand the companies’ AI services and for cloud customers who want to leverage the GPU-accelerated hardware for their own applications. Oracle had previously announced at GTC in Europe that it would be providing HGX-2 hardware for its cloud customers.
Support for the platform by system providers also got a big boost this week. At the same GTC conference, it was revealed that Inspur is launching its AI Super-Server AGX-5, becoming the first Chinese OEM to build a system based on the HGX-2. Huawei, Lenovo, and Sugon also disclosed that they have become NVIDIA HGX-2 cloud server platform partners. That news comes on the heels of announcements in May from Foxconn, Inventec, Quanta and Wistron, four of the world’s largest ODMs, that will be offering HGX-2-based systems before the end of the year.
The demand for such systems is related to its raw computing capacity and its ability to make that capacity act as a unified accelerator. A single HGX-2 node can deliver two petaflops of 16/32-bit Tensor Core performance for AI workloads and 125 teraflops of 64-bit performance for more traditional HPC. To support that computation, it provides half a terabyte of memory and 16 TB/second of aggregate memory bandwidth. According to NVIDIA, an HGX-2 system can run machine learning workloads nearly 550 times faster, deep learning workloads nearly 300 times faster, and HPC workloads nearly 160 times faster than a dual-socket CPU-powered server.
Of course, you’re going to pay a good bit of money for that privilege. The DGX-2, NVIDIA’s own HGX-2 box that includes 30 TB of NVMe storage, retails for $400K. HGX-2 implementations from OEMs, and especially ODMs, are likely to be a significantly less expensive.
Although most of HGX-2-based machinery will be applied to AI, some of these systems will get a workout from science and engineering simulations, which will take advantage of the hardware’s 64-bit capabilities. Recent DGX-2 deployments in the US at Brookhaven National Lab, Oak Ridge National Lab, Pacific Northwest National Laboratory, and Sandia National Laboratories are likely to see a significant amount for HPC action.
The potential for more of this kind of work also exists in the cloud deployments at Oracle, Baidu, and Tencent, since some customers with GPU-capable HPC codes could be drawn to performance-boost afforded by NVSwitch. Giving more customers accessibility to these systems via the cloud could create something of a virtuous circle, where more applications exposed to these GPU-dense environments creates additional demand for the underlying platform. Which is why we should expect to see more cloud partnerships around HGX-2 in the future.