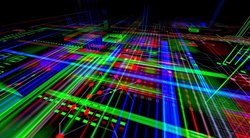
June 13, 2017
By: Michael Feldman
A research team at the Massachusetts Institute of Technology (MIT) has come up with a novel approach to deep learning that uses a nanophotonic processor, which they claim can vastly improve the performance and energy efficiency for processing artificial neural networks.
The research work, documented in a paper published this week in the journal Nature Photonics, employs a nanophotonic processor comprised of “a cascaded array of 56 programmable Mach–Zehnder interferometers in a silicon photonic integrated circuit.” The researchers demonstrated the utility of the approach with a vowel recognition application.
Optical computers have been conceived before, but are usually aimed at more general-purpose processing. In this case, the researchers have narrowed the application domain considerably. Not only have they restricted their approach to deep learning, they have further limited this initial work to inferencing of neural networks, rather than the more computationally demanding process of training.
The way it works is through the interactions of beams of light produced by the chip. This generates the interference patterns that produce the results for a given matrix multiplication operation. Essentially, they are using the interaction between the photons to perform low-level deep learning computations.
Since little power is needed to drive the light, the researchers estimate that the matrix multiplication operations implemented in photonics will use less than one-thousandth as much energy as conventional processors like GPUs or CPUs. And, since no electrons are involved, it executes these operations much faster.
"This chip, once you tune it, can carry out matrix multiplication with, in principle, zero energy, almost instantly," says Marin Soljacic, one of the researchers on the MIT team.
In this case, tuning the chip consists of modifying the array of 56 waveguides in such a way that produces a specific interference pattern, which corresponds to a particular matrix operation. This provides the programmability.
To demonstrate the approach, the researchers implemented a simple neural network that would recognize four basic vowel sounds. With this single chip, they were able to achieve a 77 percent accuracy level. That doesn’t quite match the 90 percent accuracy of conventional deep learning system, but the researchers believe they can scale up the platform fairly easily to deliver better results.
If it pans out, such a highly efficient inferencing system would be ideal for situations where power is limited, such as self-driving cars, flying drones, or mobile consumer devices. At this point though, it’s just a research project. Practical commercial implementations will require a lot more development effort.