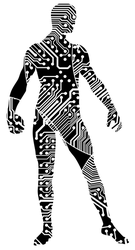
May 3, 2017
By: Michael Feldman
Diagnosing disease is one of the more labor-intensive aspects of the healthcare system. It also happens to be one that is particularly well-suited to being performed by machine learning algorithms. While work in this area is in its early stages, the technology is evolving rapidly and appears poised to transform diagnostic medicine.
Thanks largely to the huge volumes of data collected from patients, medical diagnostics is an ideal domain for machine learning. Much of the diagnostic data is image-based, such as X-rays, MRI scans, and ultrasound imagery, but can also include things like genomic profiles, epidemiological data, blood tests, biopsy results, and even medical research papers. As a result, there is a wealth of data available for training neural networks and for other machine learning techniques.
There is also a critical need for quick, accurate diagnoses. The healthcare system cannot always provide this, at least not everywhere, or at a price point that is within reach of all patients. Machine learning promises to cut costs dramatically, while providing something approaching real-time diagnostics. And in an increasing number of instances, the software can deliver a more accurate diagnosis than that of a trained physician.
One very recent example of this is an application developed by South Korean researchers at Cheonan Public Health Center and Kyong Hwan Jin at the Korea Advanced Institute of Science and Technology. According to a recent report in MIT Technology Review, Hongyoon Choi and Hwan Jin built a deep convolutional neural network (CNN) that is able to accurately identify people who will develop Alzheimer’s disease within three years, based soley on a PET (positron emission tomography) brain scan.
Using a dataset of brain images of people with full-blown Alzheimer’s, those with mild cognitive impairment (MCI), and those with MCI that subsequently developed Alzheimer’s, Hongyoon and Kyong were able to train a CNN that could predict the disease with an accuracy of 84 percent. That’s much better than what a trained diagnostician could manage to do.
Early diagnosis is important in Alzheimer’s since treatment can often slow progression of the disease if it’s undertaken before symptoms become too severe. In the US, where Alzheimer’s is the sixth leading cause of death, the cost of care is expected in be $259 billion in 2017. And that’s expected to skyrocket to $1.1 trillion by 2050.
Early diagnosis is key to reducing costs and improving outcomes for most diseases. For example, with skin cancer, the five-year survival rate is 97 percent if it’s detected in its earlier stages, but drops to 14 percent if it’s detected in its latest stages.
Such a disparity makes the deep learning algorithm developed by Stanford researchers, a potential life-saver. Sebastian Thrun, a professor at the Stanford Artificial Intelligence Laboratory, led a team that developed a convolutional neural network model that could identify both keratinocyte carcinomas and malignant melanomas as well as trained dermatologists. According to their subsequent paper published in Nature, their CNN “achieves performance on par with all tested experts across both tasks, demonstrating an artificial intelligence capable of classifying skin cancer with a level of competence comparable to dermatologists.”
This particular application was trained with 130,000 images of various skin lesions, which proved to be plenty for the software to achieve a high level of accuracy. And because the algorithm relies on skin imagery alone, anyone with a smartphone with a decent camera could potentially access this application and pre-screen themselves at no cost.
The same goes for early detection of heart disease, which is the number one cause of death worldwide. A University of Nottingham study found that a set of machine learning algorithms assessing cardiovascular risk outperformed the standard (non-machine-learning) algorithm established by the American College of Cardiology (ACC).
In this case, the input dataset was 378,256 patient records, which was applied to four algorithms based on different machine learning techniques: random forest, logistic regression, gradient boosting machines, and neural networks. The goal was to predict an individual’s chance for a heart attack or stroke with a 10-year time horizon. The algorithms’ predictions were compared against the prediction made by the ACC’s established algorithm.
The results showed that the all four machine learning algorithms were better at predicting cardiovascular disease than the ACC algorithm, with the neural network technique performing the best. "Our study shows that artificial intelligence could significantly help in the fight against it by improving the number of patients accurately identified as being at high risk and allowing for early intervention by doctors to prevent serious events like cardiac arrest and stroke,” said Dr. Stephen Weng, from the university's NIHR School for Primary Care Research.
Other recent work with machine learning models include those that can diagnose or suggest treatment for breast cancer, lung cancer, brain tumors, and even suicide. In all cases, the software’s predictive capacity was either as good as or better than that of trained doctors. These examples and the ones described above in more detail above, were all from reports published within the last few months. And these are by no means exhaustive. In any given week, there is at least one study or research project that describes a new application in machine learning-mediated medical diagnostics.
Some of these applications are now moving from the academic arena into clinical practice. In the US, the Food and Drug Administration (FDA), is already approving machine learning applications for these tasks. In January, Arterys, a cloud-based medical imaging company, received such approval for its Cardio DL application, which uses a deep learning model for cardiac MRI-related analysis.
Given the pressure to cut healthcare costs and improve access, more such approvals are surely on the way. It won’t happen overnight of course. The software must prove itself to FDA and other health agencies around the world, which are developing strict guidelines for these applications. Beyond that, very few practicing physicians are aware of machine learning as a potential diagnostic tool, so acceptance will require education and, in some cases, retraining. Nonetheless, such hurdles will eventually be overcome. The prospect of healthcare that is less expensive, more accessible, and of higher quality, will drive this technology into the mainstream.