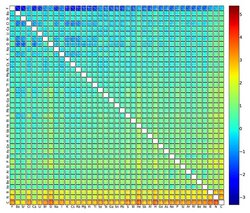
Sept. 21, 2016
By: Michael Feldman
Researchers at the University of Basel in Switzerland have used machine learning to predict the thermodynamic characteristics of 90 new mineral compounds with potential commercial use. The machine learning models were able to predict the chemical stability of all possible iterations of a particular type of class of crystals several orders of magnitude faster than if the researchers had relied on quantum mechanical calculations.
The class of compounds in this case was elpasolite, a crystalline material that in nature is made up of four chemical elements: sodium, potassium, aluminum, and fluorine. Some elpasolites emit light when exposed to ionic radiation, which makes them candidates for scintillators, a material that can be used in devices like radiation detectors, CT scanners, and various types of medical diagnostic equipment. Elpasolites could also have applications in electronic equipment, since it can serve as a conductor, semiconductor or insulator, depending upon its specific composition.
Using a machine learning model, Felix Faber, a doctoral at the University of Basel’s Department of Chemistry, was able to compute the characteristics of about two million different elpasolite compounds, based on different configurations of its four elements. To achieve that, Faber first employed brute-force quantum mechanics to predict the characteristics of thousands of randomly determined crystals, and then used those to train his statistical machine learning model. Apparently, the accuracy of the resulting model was “equivalent to that of standard quantum mechanical approaches.”
The advantage of the machine learning approach was speed. According to the university's press release, the computational power required was just a fraction of what would have been required for calculations using quantum mechanics:
Within a day, the [machine learning] model was able to predict the formation energy – an indicator of chemical stability – of all two million elpasolite crystals that theoretically can be obtained from the main group elements of the periodic table. In contrast, performance of the calculations by quantum mechanical means would have taken a supercomputer more than 20 million hours.
Of the two million compounds characterized, 90 were thermodynamically stable, and could therefore be regarded as new materials. Some of the newly discovered compounds exhibited “exotic electronic characteristics and unusual compositions,” suggesting possible consideration for commercial use.