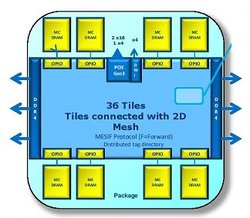
Aug. 24, 2017
By: Michael Feldman
At the Hot Chips conference this week, Intel lifted the curtain a little higher on Knights Mill, a Xeon Phi processor tweaked for machine learning applications.
As part of Intel’s multi-pronged approach to AI, Knights Mill represents the chipmaker’s first Xeon Phi offering aimed exclusively at the machine learning market, specifically for the training of deep neural networks. For the inferencing side of deep learning, Intel points to its Altera-based FPGA products, which are being used extensively by Microsoft in its Azure cloud (for both AI and network acceleration). Intel is also developing other machine learning products for training work, which will be derived from the Nervana technology the company acquired last year. These will include a Lake Crest coprocessor, and, further down the road, a standalone Knights Crest processor.
In the meantime, it’s will be up to Knights Mill to fill the gap between the current Knights Landing processor, a Xeon Phi chip designed for HPC work, and the future Nervana-based products. In this case, Knights Mill will inherit most of its design from Knights Landing, the most obvious modification being the amount of silicon devoted to lower precision math – the kind best suited for crunching on neural networks.
Essentially, Knights Mill replaces the two large double precision /single precision floating point (64-bit/32-bit) ports on Knights Landing’s vector processing unit (VPU), with one smaller double precision port and four Vector Neural Network Instruction (VNNI) ports. The latter supports single precision floating point and mixed precision integers (16-bit input/32-bit output). As such, it looks to be Intel’s version of a tensor processing unit, which has its counterpart in the Tensor Cores on NVIDIA’s new V100 GPU. That one, though, sticks with the more traditional 16/32-bit floating point math.
The end result is that compared to Knights Landing, Knights Mill will provide half the double precision floating point performance, twice the single precision floating point performance. With the added VNNI integer support in the VPU (256 ops/cycle), Intel is claiming Knights Mill will deliver up to four times the performance for deep learning applications.
The use of integer units to beef up deep learning performance is somewhat unconventional, since most of these applications are used to employing floating point math. Intel, however, maintains that floating point offers little advantage in regard to accuracy, and is significantly more computationally expensive. Whether this tradeoff pans out or not remains to be seen.
Knights Mill will also support 16 GB of MCDRAM, Intel’s version of on-package high bandwidth memory assembled in a 3D stack, as well as 6 channels of DDR4 memory. From the graphic they presented at Hot Chips (above), the design appears to support 72 cores, at least for this particular configuration. Give the 256 ops/cycle value for the VPU, that would mean Knights Mill could deliver more than 27 teraops of deep learning performance for say, a 1.5 GHz processor.
We’ll find out what actual performance can be delivered once Intel starts cranking out the chips. Knights Mill is scheduled for launch in Q4 of this year.