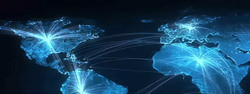
Jan. 10, 2017
By: Michael Feldman
Like many tech companies, IBM is starting the new year by making a few predictions. One of them has to do with a software concept they call a “macroscope,” a software technology that can be used to analyze the complexities of the physical world. IBM predicts that within five years, such technology will “help us understand the Earth’s complexity in infinite detail.”
Hyperbole aside, the goal is to better manage world’s resources and commercial endeavors that use those resources by applying machine learning algorithms across an array of data sources. That includes geospatial data (weather, soil, water, etc.) as well as data about economic, social and political conditions. The idea is to manage things like food, water and energy with much greater precision. All of this dovetails rather nicely under IBM’s “Smarter Planet” mantra.
Image: IBM
The work to develop this macroscope technology is being done by a team of scientists in IBM Research’s Physical Analytics group. Hendrik Hamann, the group’s research manager describes the work as an intersection between big data and physics – a field that he refers to as "physical analytics." That’s probably a more useful term than macroscopes, a metaphorical reference to something intended to measure very large things. It’s the analytics capability, rather than the measurement aspect that is at the heart of the technology.
“My team’s expertise in physical models, machine-learning, sensors, data curation and big data technologies has been put to use in applications dealing with renewable energy, precision agriculture and energy management,” writes Hamann. “We are now leading the company’s research in the quickly developing area of the Internet of Things (IoT), an extension of the classical internet of computers to any physical object.”
Dealing with IoT data is a massive challenge. According to Gartner’s estimates, in 2015 there were more than 6.4 billion IoT devices in service, and those were being added to at the rate of about 5.5 million new devices each day. That works out to about a 30 percent increase year-over-year. Given the amount of streaming data that represents (tens of exabytes per month), no single system is capable storing it, much less analyzing it.
However, managing subsets of this global data is certainly feasible for specific problems. An initial implementation of the macroscope technology is IBM’s Physical Analytics Integrated Data Repository and Services (PAIRS), a platform that enables assembly, retrieval, and analysis of geospatial datasets. PAIRS ingests raw data from a variety of public and private repositories. Those include such sources as NASA, the US Department of Agriculture, NOAA, and the Met Office, as well as other internet sites. Unlike a traditional geographic information system (GIS), where data is scattered between different sources, leaving it up to the user to deal with the different formats and the data management, PAIRS offers a curated data-as-a-service capability.
PAIRS dynamically monitors web pages and FTP sites for new data, which is then downloaded, filtered, and remapped into its internal data store. At the center of PAIRS is a Hadoop/Hbase server cluster that is capable of storing and analyzing petabytes of data. The system uses a data indexing method which “result in spatially and temporally linked data layers, both for data from 2D grids (e.g. satellite images, weather, soil, land use, etc.) and from point locations (e.g. social media data, measurements from distributed sensor networks etc.).” An API is provided for applications to query the database.
The inspiration for the technology began with a precision agriculture project that IBM researchers got involved with in 2012. Gallo Winery was looking to optimize its use of irrigation water in its vineyards, which turns out to be a big expense for the 12,000 acres of wine grapes it maintains in California. Using real-time meteorological and soil sensor data, along with satellite imagery and historical weather records, IBM researchers were able to devise software that could calculate optimal water usage across a given vineyard. Irrigation was controlled remotely via IBM’s cloud service. After three years, they achieved a 26 percent increase in grape yield with 15 percent more efficient use of water in their test plots. Simultaneously, grape quality improved by 50 percent.
IBM Research’s Physical Analytics group wants to refine and generalize these precision agriculture techniques for global deployment. And not just for irrigation. By applying the proper machine learning techniques, choice of crop variety, planting time, and fertilizer regime can all be optimized, and these are especially critical where food supply and security are pressing issues.
Hamann says the biggest hurdle is curation. According to him data scientists can spend 80 to 90 percent of their time cleaning, indexing and formatting the data. He concludes: “At the heart of our vision and development of a platform for collecting, curating and searching global data by space and time, is a set of technologies that include new indexing schemes for data from the physical world, smart cognitive data curation, parallel processing, and both large scale and physics-inspired machine-learning.”