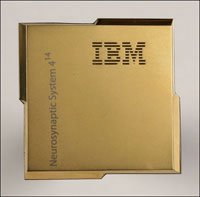
Sept. 23, 2016
By: Michael Feldman
TrueNorth, IBM’s brain-like microprocessor, has been found to be exceptionally proficient at inference work for deep neural networks. In particular, the chip has demonstrated it’s especially good at image recognition, being able accurately classify such data much more efficiently, from an energy perspective, than traditional processor architectures, suggesting new applications in mobile computing, IoT, robotics, autonomous cars, and HPC.
An IBM Research blog post about TrueNorth’s foray into deep learning, noted that the classification accuracy demonstrated by the system approached that of state-of-the-art implementations, and not just for image recognition, but for speech recognition as well. “The new milestone provides a palpable proof-of-concept that the efficiency of brain-inspired computing can be merged with the effectiveness of deep learning, paving the path towards a new generation of cognitive computing spanning mobile, cloud, and supercomputers,” said IBM Fellow Dharmendra Modha, chief scientist, Brain-inspired Computing, IBM Research.
Specifically, TrueNorth was able to classify images at between 1,200 and 2,600 frames per second (fps), while drawing just 25 to 275 milliwatts of power. That works out to about 6000 fps per watt, which would allow a low-power device to classify images in real-time from dozens of standard TV video feeds simultaneously. For the sake of comparison, NVIDIA’s latest purpose-built inferencing GPU, the Tesla P4, can classify images at about 160 images per second per watt using AlexNet.
Training the model was performed with conventional GPUs, using functions from the MatConvNet toolbox, along with some customizations. On the inferencing side, the researchers developed an algorithm that exploited the low precision synapses and spiking neuron design of the TrueNorth processor. For the demonstration, eight image and audio benchmarks were employed, using five configurations of 0.5, 1, 2, 4, or 8 of the chips.
In retrospect, maybe it shouldn’t come as a surprise that a neuromorphic architecture would be so adept at neural networks. But at the time TrueNorth was developed in 2011, the modern approach to convolutional networks was just getting traction. So while there was no design consideration for such a model, it became apparent to the researchers that the underlying architecture was well-suited to the application model once the appropriate algorithm was devised.
Beside embedded deep learning applications on smartphones, robots and such, a TrueNorth-accelerated system could also be the basis of a scalable approach for deep learning inferencing in hyperscale or HPC datacenters. A possible testbed for such research already exists at the Department of Energy’s Lawrence Livermore National Laboratory (LLNL). In March, the lab installed a 16-chip TrueNorth array, known as NS16e, which was designed to explore scalable neuromorphic computing.
One of the applications LLNL is currently looking at is how to use the processor to identify cars from overhead imagery, like for example, from video captured by airborne drones. Another use case explores the detection of defects in additive manufacturing (industrial 3D printing). A third application area being researched is how to use the platform to supervise physics simulations in industrial designs.
The deep learning demonstration indicates that the architecture has an even broader scope though, and one that dovetails nicely with commercial developments in AI. Says Modha: “This work finally proves that the neuromorphic approach can be efficiently used in real-world applications and has significant advantages over conventional digital devices when energy consumption is considered.”