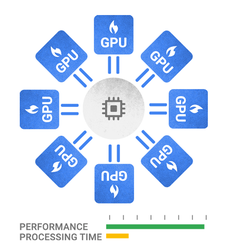
Feb. 21, 2017
By: Michael Feldman
The largest Internet company on the planet has made GPU computing available in its public cloud. Google announced this week that it has added the NVIDIA Tesla K80 to its cloud offering, with more graphics processor options on the way. The search giant follows Amazon, Microsoft and others into the GPU rental business.
According to a blog posted Tuesday, a user can attach up to four K80 boards, each of which houses two Kepler-generation GK210 GPUs and a total of 24GB of GDDR5 memory. The K80 delivers 2.9 teraflops of double precision performance or 8.73 teraflops of single precision performance, the latter of which is the more relevant metric for deep learning applications. Since we’re talking about a utility computing environment here, a user may choose to rent just a single GPU (half a K80 board) for their application.
The initial service is mainly aimed at AI customers, but other HPC users should take note as well. Although Google has singled out deep learning as a key application category, the company is also targeting other high performance computing applications, including, computational chemistry, seismic analysis, fluid dynamics, molecular modeling, genomics, computational finance, physics simulations, high performance data analysis, video rendering, and visualization
Google’s interest in positioning its GPU offering to deep learning is partially the result of the in-house expertise and software the company has built in this area over the last several years. The new cloud-based GPU instance have been integrated with Google’s Cloud Machine Learning (Cloud ML), a set of tools for building and managing deep learning codes. Cloud ML uses the TensorFlow deep learning framework, another Google invention, but which is now maintained as an open source project. Cloud ML helps users employ multiple GPUs in a distributed manner so that the applications can be scaled up, the idea being to speed execution.
The Tesla K80 instance is initially available as a public beta release in the Eastern US, Eastern Asia and Western Europe. Initial pricing is $0.70 per GPU/hour in the US, and $0.77 elsewhere. However, that doesn’t include any host processors or memory. Depending on what you want, that can add as little as $0.05 per hour (for one core and 3.75 GB of memory), all the way up to more than $2 per hour (for 32 cores and 208 GB of memory). For a more reasonable configuration, say four host cores and 15 GB of memory, an additional $0.20 per hour would be charged.
That would make it roughly equivalent to the GPU instance pricing on Amazon EC2 and Microsoft Azure, which include a handful of CPU cores and memory by default. Both of those companies, which announced GPU instances for their respective clouds in Q4 2016, have set their pricing at $0.90 per GPU/hour. For users willing to make a three-year commitment, Amazon will cut the cost to $0.425 per GPU/hour via its “reserved instance” pricing.
IBM’s SoftLayer cloud also has a number of GPU options, but they rent out complete servers rather than individual graphics processors. A server with a dual-GPU Tesla K80, two eight-core Intel Xeon CPUs, 128 GB of RAM, and a couple of 800GB SSDs will cost $5.30/hour. Other K80 server configurations are available for longer terms, starting at $1,359/month.
At this point, HPC cloud specialist Nimbix has what is probably the best pricing for renting GPU cycles. They’re offering a K80-equipped server – so two GPUs – with four host cores and 32 GB of main memory for $1.06/hour. That’s substantially less expensive than any others cloud providers mentioned, assuming your application can utilize more than a single GPU. Nimbix is also the only cloud provider that currently offers a Tesla P100 server configuration, although that will cost you $4.95 per hour.
Even though the initial GPU offering from Google is confined to the Tesla K80 board, the company is promising NVIDIA Tesla P100 and AMD FirePro configuration are “coming soon.” The specific AMD device is likely to be the FirePro S9300 x2, a dual-GPU board that offers up to 13.9 teraflops of single precision performance. When Google previewed its accelerator rollout last November, it implied the FirePro S9300 x2 would be aimed at cloud customers interested in GPU-based remote workstations. The P100 is NVIDIA’s flagship Tesla GPU, delivering 5.3 or 10.6 teraflops of double or single precision performance, respectively.
At this point, Google is in third place in the fast-growing public cloud space, trailing Amazon and Microsoft, in that order. Adding a GPU option is not likely to change that, but it does illustrate that graphics processor-based acceleration is continuing to spread across the IT datacenter landscape. Whereas once GPU acceleration was confined to HPC, with the advent of hyperscale-based machine learning, it quickly became standard equipment for hyperscale web companies involved in training neural networks. Now that more enterprise customers are looking to mine their own data for machine learning purpose, the GPU is getting additional attention. And for traditional HPC, many of the more popular software packages have already been ported to GPUs.
This all might be good news for Google, but it’s even better news for NVIDIA, and to a lesser extent AMD, which still stands to benefit from the GPU computing boom despite the company’s less cohesive strategy. NVIDIA just announced a record revenue of 6.9 billion for fiscal 2017, driven, in part, by the Tesla datacenter business. That can only get better as GPU availability in the cloud becomes more widespread.